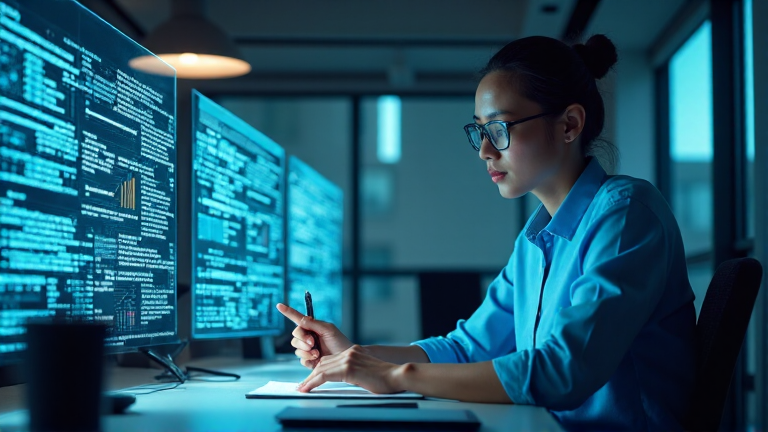
Unlocking Business Insights: The Power of Prompt Engineering
In today's rapidly evolving business landscape, artificial intelligence (AI) is a game-changer, enabling companies to mine valuable insights from vast datasets. Business analysts stand at the forefront of this transformation, bridging raw data with strategic decision-making. A new skill indispensable to their arsenal is prompt engineering—the refined art of crafting precise inputs to elicit optimal results from AI models. Mastering this skill not only enhances the value they bring but also ensures they remain indispensable in an era driven by technological advancement.
Understanding Prompt Engineering
Prompt engineering involves the development of inputs, known as "prompts," designed to elicit specific, accurate, and actionable responses from AI models. Large language models (LLMs), such as GPT-4, possess tremendous capabilities, yet require clear and structured prompts to perform effectively. A well-crafted prompt directs the AI to focus on relevant data, deliver responses in designated formats, or prioritize certain outcomes.
Consider these prompts: "What are the current trends in how customers act?" versus "Summarize the top three purchasing trends among customers aged 25 to 40, including seasonal patterns, based on recent sales data." The latter provides clarity, focus, and context, ensuring the AI aligns its output with the analyst's objectives.
Why Business Analysts Should Prioritize Prompt Engineering
As AI tools like ChatGPT, Claude, and Gemini integrate further into business workflows, business analysts recognize that mastering prompt engineering can significantly enhance productivity. It allows for:
- Improved Decision-Making: Tightly constructed prompts extract valuable insights, empowering organizations to make data-driven decisions aligned with their goals.
- Time Efficiency: Clear prompts reduce the need for repeated inquiries, expediting the insight-gathering process.
- Custom Insights: Refined prompts provide results tailored to specific business contexts, ensuring the relevance and utility of the outcomes.
- Maximized AI Potential: Effective prompts allow AI models to fully harness their functionalities, transforming them into robust tools rather than mere answer generators.
Best Practices for Business Analysts in Prompt Engineering
To fully leverage prompt engineering, business analysts should adhere to several best practices:
- Clarity and Specificity: Clearly define objectives and include essential data. Vague prompts often lead to unclear or incomplete outputs.
- Iterative Refinement: Test various prompts to determine the most effective wording and structure.
- Utilize Contextual Cues: Provide the AI with background knowledge or frameworks to guide its responses.
- Incorporate Examples: Examples within prompts assist AI in understanding the expected style or detail level.
- Foster Interactions: Engage with follow-up questions to deepen insights or explore tangential ideas, facilitating dynamic exploration of topics.
Navigating Impediments in Prompt Engineering
Despite the advantages AI offers in promoting innovation, challenges persist:
- Learning Curve: Crafting effective prompts demands practice and a nuanced understanding of AI model capabilities and limitations.
- Bias and Fairness: Poorly designed prompts may unintentionally introduce bias, leading to skewed insights. Analysts must ensure prompts promote fairness and inclusivity.
- Dependence on Data Quality: The quality of AI outputs heavily relies on the data quality employed. Analysts should verify data accuracy and reliability prior to using AI models.
- Adapting to Evolution: As AI models evolve, prompt engineering techniques must evolve concurrently. Continuous learning is essential to remain effective.
Advanced Prompt Engineering Techniques for Business Analysts
Here are some validated prompt engineering strategies business analysts can employ:
Technique 1: Expert Persona Simulation
To obtain more relevant AI responses, instruct the model to assume a specific role, which aids in simulating expertise in a given domain.
- Prompt Example: "You are a seasoned Business Analyst with expertise in [industry/domain]. Your task is to [describe the request]."
- Use Case: Structuring business requirements for a new ERP system.
- Example: "You are an experienced Business Analyst in ERP implementation. Provide a structured business requirements document outlining key functional and non-functional requirements for an ERP system in a manufacturing company."
Technique 2: Reverse Engineering Communication Styles
Reverse engineering the communication styles of leading consulting firms allows analysts to emulate high-quality reporting without direct imitation.
- Prompt Example: "Describe the key elements of [expert/company]’s business analysis reports in bullet points. Then, generate a [deliverable] in that style."
- Use Case: Structuring a competitive analysis report similar to McKinsey or Gartner.
- Example: "Describe the key elements of a Gartner-style market analysis report in bullet points. Then, generate a competitive analysis report on AI adoption in the financial sector using that style."
Technique 3: Emotion-Driven AI Engagement
Framing tasks emotionally can lead to more thorough AI responses, especially in executive reports or stakeholder communications.
- Prompt Example: "Help me [task]. Please make sure [key attribute]. This is critical for [impact or goal]."
- Use Case: Justifying ROI for a new data analytics tool to executives.
- Example: "Help me draft a compelling executive summary on the ROI of implementing a new data analytics tool. Ensure the message is data-driven, persuasive, and addresses cost-benefit analysis. This is critical for securing approval from senior leadership."
Technique 4: Pattern Learning through Examples
Providing examples helps AI generate structured responses when following specific formats.
- Prompt Example: "Here are some examples of [task]. Generate a [new instance] in the same format."
- Use Case: Generating consistent user stories.
- Example: "Here are two examples of user stories from our backlog: As a finance analyst, I want to automate expense tracking so I can reduce manual errors. As a sales manager, I want a dashboard summarizing key KPIs so I can track performance in real time. Now, generate a user story for integrating machine learning-based fraud detection."
Technique 5: Generating Synthetic Data for Analysis
When actual data is limited, AI can create realistic test cases or scenarios for evaluation and decision-making.
- Prompt Example: "Generate ten examples of [scenario] for [context]."
- Use Case: Creating sample survey responses for stakeholder assessments.
- Example: "Generate ten sample stakeholder responses on the challenges of adopting cloud-based enterprise solutions."
The Future of Prompt Engineering in Business Analysis
Integrating prompt engineering into business analysis heralds a new era of AI-driven decision-making. As AI models advance, prompt engineering will eventually:
- Provide Dynamic Decision Support: Offering insights tailored to changing business environments in real time.
- Enable Personalized AI Assistants: Advanced models will understand organizational intricacies, anticipating analytical needs.
- Facilitate Scalable Analytics: Streamlining AI capabilities so non-technical stakeholders can equally leverage AI insights.
As AI continues to evolve, combining prompt engineering with business analysis is set to spur innovation, empower organizations, and influence the trajectory of data-driven strategies. Just as mastering communication fosters human collaboration, adeptness in prompt engineering amplifies AI's efficacy in business analysis. Through these techniques, analysts can: - Derive richer insights from AI tools - Automate documentation with greater efficiency - Elevate the quality of data analysis and reporting - Communicate more persuasively with stakeholders
Note: This publication was rewritten using AI. The content was based on the original source linked above.