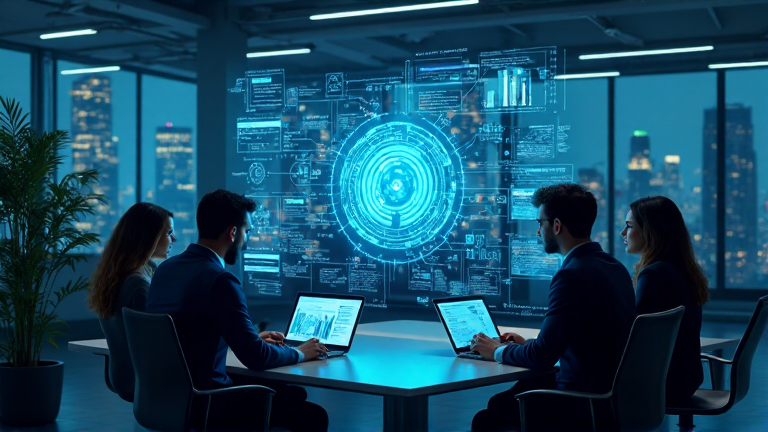
Navigating AI Success: A Holistic Framework for Businesses
In an era where almost every system boasts integration with artificial intelligence, adopting AI necessitates a strategic and comprehensive approach to realize its true value. Ajay Kumar, CEO of SLK Software, emphasizes the importance of a holistic framework that enables enterprises to tackle complex business challenges effectively.
The Significance of a Holistic Approach in AI Adoption
Implementing AI is a multifaceted process affecting various organizational domains, from marketing to operations. A holistic strategy ensures alignment of AI endeavors across these departments, optimizing efficiency and promoting synergy. Beyond technical interventions, embracing AI requires significant cultural shifts with a focus on training and supporting employees to adopt new technologies. Addressing mindset changes and fostering collaboration are essential.
Ethical considerations, such as data privacy and potential biases, are other elements demanding scrutiny in AI adoption. A holistic stance aids in developing responsible frameworks, enhancing stakeholder trust. The reliance of AI on data underscores the necessity for rigorous data quality assessment and governance to build dependable systems.
Furthermore, adaptability and scalability of AI initiatives ensure future readiness as technology and market conditions evolve. Including diverse stakeholder insights—from executives to end-users—ensures comprehensive engagement and encourages buy-in crucial for successful implementation. Defining success metrics that align with both technical performance and business objectives is also pivotal.
Understanding the AI Lifecycle and Its Importance
The AI lifecycle constitutes a structured blueprint guiding AI system development, deployment, and maintenance. It typically encompasses:
- Business Understanding or Problem Definition: Clearly defining the problem AI aims to solve and its strategic business value.
- Data Understanding and Collection: Acquiring quality data vital for AI model training and evaluating data accessibility.
- Data Preparation: Data cleansing and feature engineering to distill meaningful attributes, ensuring project feasibility.
- Model Selection: Choosing suitable algorithms and models based on defined problems and data characteristics.
- Modelling/Training: Training models to interpret data effectively, iterating to meet business objectives.
- Evaluation: Assessing model performance using metrics like accuracy and precision.
- Deployment: Integrating AI models into existing business processes.
- Solution Monitoring: Continuous performance monitoring and adjustments to guarantee reliability.
This lifecycle aids organizations in managing AI project complexities, fostering iterative model improvement, and aligning initiatives with corporate goals.
Areas Where AI Drives Impact
AI profoundly transforms various business sectors:
- Customer Service: Enhances interactions through AI chatbots offering instant support.
- Marketing: Analyzes consumer behavior for personalized campaigns.
- Sales: Employs predictive analytics for lead identification and conversion.
- Operations: Optimizes supply chains and logistics.
- Human Resources: Automates recruitment and personalizes training.
- Finance: Facilitates fraud detection and financial reporting.
- Healthcare: Assists in diagnostics and patient monitoring.
Overall, AI propels efficiency, innovation, and competitive advantage.
Risks and Challenges in AI Modernization
Modernizing processes with AI introduces several challenges:
- Data Availability and Quality Issues: Inadequate or synthetic data may not represent real-world scenarios.
- Integration Challenges: Legacy systems lacking APIs complicate technical integration.
- Security Concerns: Susceptibility to cyber threats and adversarial attacks risks data integrity.
- BCP Situations: Over-reliance on AI can complicate operations if systems fail.
Measuring AI Success
Metrics for evaluating AI project success should align with business objectives and processes. These criteria can include:
- Business Impact and ROI
- Performance Metrics
- User Adoption
- Operational Efficiency
- Scalability
- Compliance and Ethical Standards
By focusing on these key metrics, organizations can ensure AI projects deliver tangible value and strategic benefits.
Note: This publication was rewritten using AI. The content was based on the original source linked above.