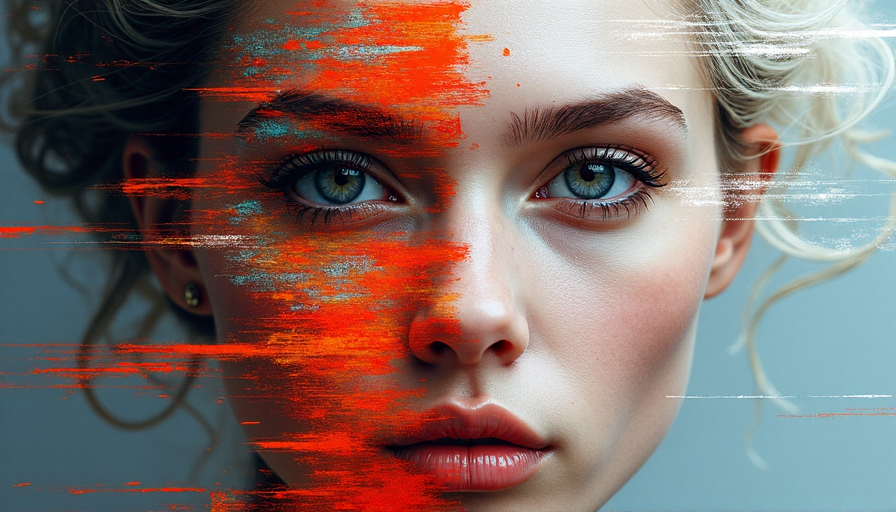
Accelerating Image Generation with HART
Researchers from MIT and NVIDIA have unveiled a breakthrough in image generation technology with the introduction of HART (Hybrid Autoregressive Transformer). Achieving the difficult balance between speed and quality, HART integrates two distinct approaches—autoregressive modeling and diffusion modeling—into one powerful, energy-efficient tool that can run on everyday devices such as laptops and smartphones.
A Tale of Two Models
Traditional diffusion models, like Stable Diffusion and DALL-E, have long been celebrated for producing highly detailed, realistic images. They work by repeatedly refining images through a lengthy process of de-noising, correct errors, and adding intricate details. Although the resulting images are stunning, the process is both slow and computationally heavy.
On the flip side, autoregressive models, which power many language tools including ChatGPT, generate images by predicting small sections or tokens. This method is fast but usually introduces errors and misses out on finer details. Recognizing the limits of each method, the research team decided to combine the best aspects of both.
The Hybrid Approach: HART in Action
HART starts by using an autoregressive model to rapidly capture the overall composition of an image. Then, it employs a small, efficient diffusion model to refine the details—essentially applying the perfect finishing touches to ensure clarity and high fidelity. This clever sequencing allows the diffusion component to work in fewer steps (around eight) compared to the typical 30-plus steps required by pure diffusion models.
This dual-stage method not only results in images that match or exceed the quality of traditional models but does so approximately nine times faster and with 31% less computation. As a result, generating a high-quality image requires significantly fewer resources and can even be accomplished on commercial laptops and smartphones.
Implications and Future Horizons
HART's innovative design opens up numerous possibilities. With the major part of processing handled by the autoregressive model—similar to the engines behind modern large language models—HART is inherently compatible with next-generation vision-language systems. This compatibility could lead to the development of unified models that excel in both visual and reasoning tasks.
Looking forward, the researchers are enthusiastic about extending HART's principles to additional media, including video generation and audio prediction. This scalability suggests that HART could play a pivotal role in training autonomous systems like self-driving cars, assisting in real-world task simulations, and even revolutionizing the gaming and design industries.
Key Takeaways:
- Hybrid Efficiency: HART leverages an autoregressive model for rapid image layout combined with a diffusion model for detailed refinement.
- Resource Friendly: Generates high-quality images on common devices with significantly lower computational expenses.
- Future-Ready: Compatible with unified vision-language models, promising advancements in multiple multimedia domains.
This groundbreaking work, which received support from institutions such as the MIT-IBM Watson AI Lab, Amazon Science Hub, and the U.S. National Science Foundation, is set to be showcased at the upcoming International Conference on Learning Representations. The collaborative effort between MIT, Tsinghua University, and NVIDIA signals a major step forward in the field of artificial intelligence and computer graphics.
Note: This publication was rewritten using AI. The content was based on the original source linked above.