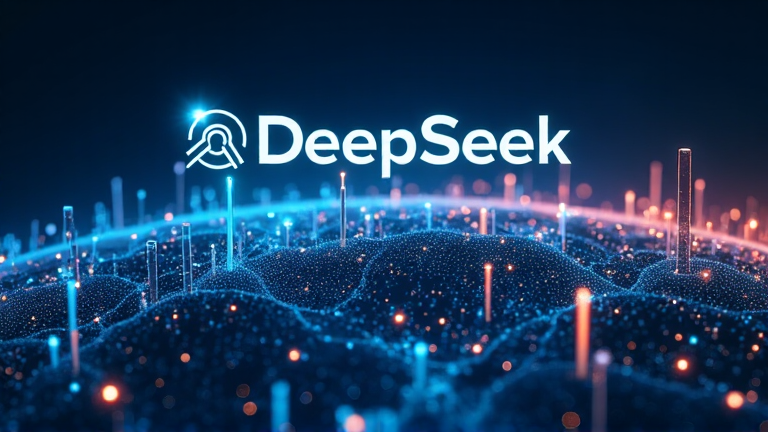
DeepSeek: A Revolution in the AI Landscape
Chinese AI firm DeepSeek has taken the tech world by storm with its pioneering advancements in artificial intelligence. With the introduction of AI models that rival top-tier products from leading U.S. companies like OpenAI and Anthropic, DeepSeek is redefining efficiency in the AI realm.
Founded in 2023, DeepSeek has demonstrated an ability to achieve remarkable results while utilizing a fraction of the financial and computational resources typical of its competitors.
Breakthroughs Unveiled
A major milestone came last week with the release of DeepSeek's "reasoning" R1 model. This development not only intrigued researchers but also caused a ripple effect among investors and prompted reactions from established AI giants. In a follow-up move on January 28, the company unveiled a model with capabilities extending to both image and text work.
The V3 Model's Impact
In December, DeepSeek introduced the V3 model, a robust large language model on par with OpenAI's GPT-4o and Anthropic's Claude 3.5. Despite the tendency of such models to make errors or invent data, they proficiently perform tasks like question answering, essay writing, and coding—often surpassing average human performance in problem-solving and math reasoning tests.
Remarkably, V3 was developed at a reported cost of just US$5.58 million, starkly lower than GPT-4's staggering development cost exceeding US$100 million. The training utilized approximately 2,000 H800 NVIDIA GPUs, considerably fewer than the up to 16,000 H100 chips often employed by others.
Introducing the R1 Model
On January 20, DeepSeek launched the R1 model, focusing on breaking down complex problems step-by-step. This approach enhances its capability in tasks necessitating contextual understanding and multilevel interrelation, such as strategic planning and reading comprehension. Based on V3's framework but refined through reinforcement learning, the R1 mirrors the performance of OpenAI's o1 model.
Furthermore, DeepSeek applied the same method to develop "reasoning" versions of smaller, open-source models operable on home computers. This release surged the interest in DeepSeek, skyrocketing the popularity of its V3-based chatbot app, while precipitating a dramatic downturn in tech stocks as investors reassess the AI sector. NVIDIA has reportedly suffered a US$600 billion devaluation.
Innovations Behind the Achievements
DeepSeek's success hinges on groundbreaking efficiency, achieving quality results with minimal resources and introducing two key techniques that are likely to be embraced by broader AI research.
-
Sparsity Technique: AI models comprise myriad parameters; however, only a fraction is active per input. Identifying these parameters is complex. DeepSeek utilized a novel method to predict and train only necessary parameters, drastically reducing training needs.
-
Data Compression: DeepSeek discovered an innovative approach to compress and swiftly access relevant data, optimizing how V3 utilizes computer memory.
Implications of DeepSeek's Advances
By releasing their models and methods under the free MIT License, DeepSeek provides open access for usage and modification—potentially impacting the profitability of proprietary AI companies but invigorating the wider AI research community.
Currently, significant AI research demands access to vast computational resources, limiting experimentation for those outside large tech enterprises. With more efficient models and techniques, barriers diminish, facilitating broader experimentation and development.
Consumers, too, might benefit from reduced AI costs, with potential for AI models to run on personal devices rather than relying on costly cloud services.
While resource-rich research entities may see limited impact from these efficiencies, it remains to be seen if DeepSeek's methods contribute to enhanced performance or chiefly emphasize efficiency.
Note: This publication was rewritten using AI. The content was based on the original source linked above.