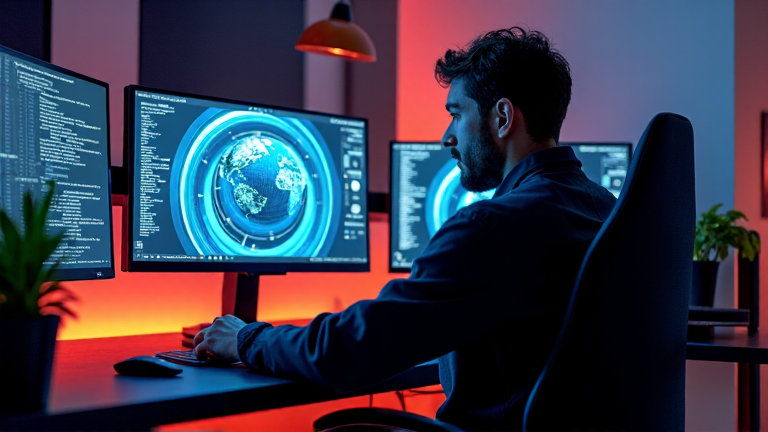
Unleashing AI Potential at Home: A Comprehensive Guide to Deepseek R1 671b
In an era where Artificial Intelligence is reshaping industries, running powerful AI models like Deepseek R1 671b locally opens a new frontier for enthusiasts and developers alike. Understanding the technical landscape of local deployment, this guide sheds light on the hurdles, breakthroughs, and the vast potentials these systems hold. By exploring open-source advancements, one can appreciate the profound impact they have on the AI ecosystem, promising a future where "garage AGI" becomes a tangible reality.
The Nuts and Bolts of Local Deepseek R1 671b Setup
Core Insights:
- Deploying the Deepseek R1 671b model locally demands formidable hardware, akin to servers endowed with 1.5TB of RAM, along with GPUs for optimization, although less costly setups might hinder efficiency.
- Performance issues such as token generation speed fluctuations necessitate meticulous system tuning to achieve optimal results.
- Despite its prowess in complex analytical tasks, the model sometimes falters on simpler queries, indicating room for enhancement.
- Deepseek R1 671b’s open-source nature spurs innovation and wider AI accessibility, stirring debates on the plausibility of "garage AGI."
- Anticipated advancements in both hardware and software point towards improved AI deployments, broadening their practical applications.
Balancing Hardware Resources: Power Meets Budget
Navigating the hardware demands of Deepseek R1 671b requires striking a delicate balance between power and affordability. Systems like the Dell R930 server, boasting 1.5TB of RAM, often cater to the model’s extensive memory cravings. Ensuring ample RAM is pivotal to maintaining system stability during inference processes.
While GPUs dominate the AI processing realm for their superior parallel processing faculties, some cost-conscious users lean towards CPUs. This choice often impairs performance due to CPUs’ less efficient matrix computation capabilities. Those opting for economical methods might resort to outdated hardware or consumer-focused GPUs, lowering expenses albeit at the cost of reduced efficiency. Crafting a cost-effective deployment strategy is essential for users with budget constraints.
Troubleshooting and Overcoming Performance Challenges
The journey of running Deepseek R1 671b locally reveals several performance bottlenecks. Token generation speed—a critical performance metric—can range considerably, influenced by factors like hardware specifics and system configuration, fluctuating between 1 to 35 tokens per second.
Mitigating these challenges requires keen system adjustments. Fine-tuning memory usage, calibrating processor affinities, or leveraging agile virtualization solutions can enhance performance. However, these adaptations often entail a process of trial and error, necessitating careful evaluation of trade-offs between resource utilization and performance when troubleshooting impediments.
Unpacking Model Capabilities: Analyzing Strengths and Limitations
The model shines in scenarios demanding complex reasoning and strategic decision-making, thanks to its aptitude in systematic problem breakdowns. It excels at scenarios requiring thoughtful resolution of ethical dilemmas or intricate problem-solving tasks.
Yet, Deepseek R1 671b doesn’t come without limitations. During evaluations, it exhibited struggles with basic processes like elementary arithmetic or straightforward inquiries. Identifying these inconsistencies helps pinpoint areas for refinement, vital for enhancing reliability across diverse applications. Understanding the model's capabilities is crucial for leveraging its strengths effectively.
The Open-Source Paradigm and Toward AGI
As an open-source venture, Deepseek R1 671b is a pivotal contributor to democratizing AI technology. By making its codebase publicly available, it empowers researchers and developers to experiment and innovate on the quest toward Artificial General Intelligence (AGI).
Its accessibility provokes discussions about "garage AGI," hypothesizing that small teams or individuals could develop advanced AI systems beyond institutional research constraints. However, challenges such as resource limitations and expertise remain formidable. Nevertheless, the open-source framework of Deepseek R1 671b extends luminary opportunities for decentralized AI advancements.
Looking Ahead: Reinforcing Efficiency and Pioneering Advances
Future improvements in hardware affordability and software refinements are crucial for making hefty models like Deepseek R1 671b more viable for on-premise operations. The landscape is shifting towards more affordable high-capacity GPUs and more refined parallel processing algorithms, easing resource demands for these models and enhancing accessibility.
Upcoming AI models, such as Quinn Vision and Janus, are anticipated to enhance upon the groundwork laid by Deepseek R1 671b, promising superior performance and augmented capabilities. These iterations will likely lead to broader adoption of high-caliber AI systems beyond conventional cloud settings.
Deploying Deepseek R1 671b locally illuminates the feasibility and challenges associated with non-cloud, large-scale AI system implementations. While demanding on the technical front, its open-source status and demonstrated brilliance make it a profound asset for advancing AI research. As technology strides forward, realizing locally deployed, cost-effective, high-performance AI—and potentially AGI—seems more within reach than ever.
Note: This publication was rewritten using AI. The content was based on the original source linked above.