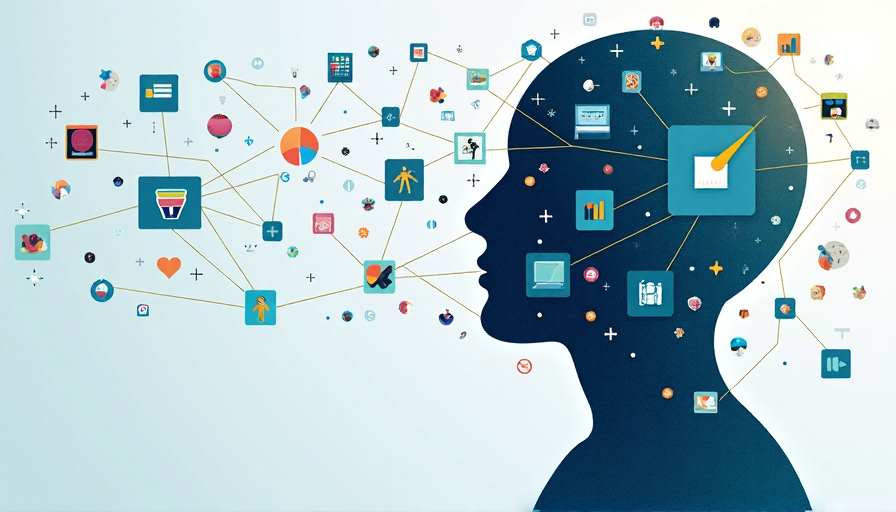
Machine Learning Advances in Diabetes Risk Prediction: Insights from NHANES Data
This article recounts a pioneering study that harnessed the power of machine learning to predict type 2 diabetes (T2DM) using patient lifestyle and anthropometric data. Based on nearly 30,000 entries from the National Health and Nutrition Examination Survey (NHANES) collected between 2007 and 2018, the research compared five different predictive algorithms with the goal of identifying individuals at risk through non-invasive means.
Overview of the Study
The study was conceived with a simple, yet compelling, question: Can easily accessible lifestyle factors such as diet and physical activity be used to predict T2DM? Researchers compiled data from over 29,000 non-pregnant adult participants, focusing on a wide range of variables that included demographics, dietary habits, body measurements, and physical activity. Rather than relying solely on laboratory tests, this research explored the potential of machine learning algorithms to offer a convenient early-warning system for diabetes risk.
Study Setting and Participants
The investigation used publicly available NHANES data, a resource known for its robust representation of the U.S. population's nutritional and health status. After handling missing entries and applying standard data quality checks, the researchers finalized a cohort of 29,509 adults. The study’s design was retrospective and cross-sectional, meaning that the data provided a snapshot into participants’ health profiles during the surveyed period.
Machine Learning Models Evaluated
Five distinct supervised learning algorithms were tested:
- Logistic Regression: A statistical method to model the relationship between variables and the probability of diabetes.
- Support Vector Machine (SVM): A classifier that finds the optimal boundary to separate diabetic from non-diabetic profiles, excelling in identifying true positives despite lower overall accuracy.
- Random Forest: An ensemble approach that creates multiple decision trees to provide a consensus prediction while highlighting feature importance.
- XGBoost: A powerful gradient boosting tool that sequentially builds decision trees, correcting errors along the way.
- CatBoost: Known for processing categorical variables effectively and offering versatility when data patterns are complex.
Advanced techniques such as grid search and 10-fold cross-validation were used to fine-tune each model's performance. Sample weighting from NHANES ensured that the outcomes reflected a representative picture of the U.S. population.
Performance Metrics
Among the five models, XGBoost emerged as the standout performer with an area under the receiver operating characteristic curve (AUC) of 0.8168. Its accuracy hovered around 85%, comparable to both logistic regression and random forest models. While most models achieved high specificity (above 97%), the SVM model was noted for its higher sensitivity of 58.57%, albeit at the cost of overall accuracy. The trade-off between sensitivity and specificity highlights the challenge in balancing the identification of true diabetic cases without sacrificing precision.
Feature Importance and Model Interpretation
Using Shapley Additive Explanations (SHAP), the study revealed that variables such as age, waist circumference, and dietary sugar intake played dominant roles in predicting diabetes risk. Notably, the analysis suggested that waist circumference might be a more robust predictor than body mass index (BMI) for this dataset. The integration of dietary and lifestyle factors into the models underscores the potential of non-invasive, data-driven strategies in early diabetes screening.
Discussion and Clinical Implications
The research not only reinforces the feasibility of using machine learning for T2DM risk prediction but also provides practical insights for healthcare practitioners. The study emphasizes that although laboratory tests remain a gold standard, models based on easy-to-obtain data can help flag individuals who should undergo further evaluation. For instance, clinicians might use these insights to initiate early lifestyle interventions for patients displaying central obesity or unhealthy dietary habits.
The findings also provoke important discussions about model selection. While XGBoost provides superior overall accuracy, the high sensitivity of SVM might be preferable in scenarios where capturing every potential case is critical. Such discussions pave the way for hybrid strategies that leverage the strengths of different models.
Strengths and Limitations
Strengths include:
- A large, nationally representative dataset that bolsters the generalizability of findings.
- A comprehensive comparison of multiple machine learning algorithms, providing insight into the best balance of accuracy and interpretability.
- Rigorous cross-validation techniques to mitigate overfitting.
Limitations involve:
- Reliance on self-reported data, which could introduce recall bias.
- A cross-sectional design that limits causal inferences and does not capture the progression of T2DM over time.
Conclusion
The study offers compelling evidence for the role of machine learning in predicting type 2 diabetes using non-invasive, routinely collected data. With XGBoost leading in predictive performance and logistic regression and random forest showing strong interpretability, the research points to multiple avenues for optimizing diabetes screening. Its findings may facilitate early intervention strategies that help mitigate the long-term complications and economic burden of T2DM. Future studies are encouraged to refine these approaches further and validate them in diverse clinical settings.
By weaving together advanced analytics and practical public health applications, this research highlights a promising step towards precision medicine in diabetes care.
Note: This publication was rewritten using AI. The content was based on the original source linked above.