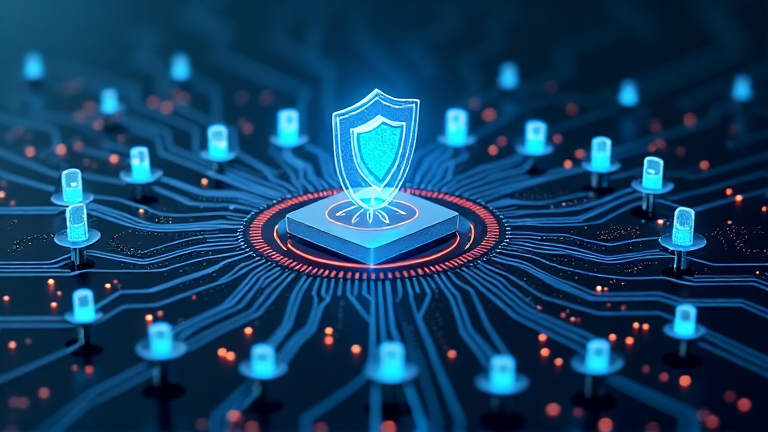
The Growing Security Challenges of AI Models
As artificial intelligence (AI) becomes deeply embedded in critical infrastructures, organizations must prioritize a multi-layered defense strategy to safeguard their systems.
AI has emerged as a transformative force across industries, revolutionizing decision-making, operational efficiency, and user experiences. However, its rapid adoption has also introduced a complex and evolving threat landscape. These risks range from traditional cybersecurity vulnerabilities to unique AI-specific challenges, such as adversarial attacks, data manipulation, and exploitation of machine learning models. These threats not only jeopardize privacy and security but also erode trust in AI systems.
With AI now integral to sectors like healthcare, finance, and national security, organizations must adopt proactive measures to identify and mitigate vulnerabilities. By doing so, they can protect their AI systems and ensure the resilience of their broader digital ecosystems.
Emerging Threats to AI Models and Users
As AI adoption grows, so do the threats targeting it. Key challenges include:
-
Trust in Digital Content: AI-generated content, such as deepfakes, is becoming increasingly difficult to distinguish from authentic material. Vulnerabilities in safeguards, like watermark manipulation, can undermine trust and spread misinformation, leading to significant social consequences.
-
Backdoors in AI Models: Open-source platforms like Hugging Face have made AI models more accessible, but they also introduce risks. For instance, the 'ShadowLogic' technique developed by HiddenLayer’s Synaptic Adversarial Intelligence (SAI) team allows attackers to implant undetectable backdoors into neural networks, compromising their integrity even after fine-tuning.
-
Integration into High-Impact Technologies: AI models, such as Google’s Gemini, are vulnerable to indirect prompt injection attacks. These can manipulate models into producing harmful outputs or even executing unauthorized API calls, emphasizing the need for robust defenses.
-
Traditional Security Vulnerabilities: Common vulnerabilities in AI infrastructure, particularly in open-source frameworks, remain a significant concern. Attackers often exploit these weaknesses, making proactive identification and mitigation essential.
-
Novel Attack Techniques: New methods, such as Knowledge Return Oriented Prompting (KROP), bypass traditional safety measures in large language models (LLMs), creating unforeseen risks. These techniques highlight the need for continuous innovation in AI security.
Staying Ahead of Adversaries
To counter these threats, researchers must anticipate adversarial tactics and uncover vulnerabilities before they are exploited. Proactive research, combined with automated tools, enables the discovery of new Common Vulnerabilities and Exposures (CVEs). Responsible disclosure of these vulnerabilities strengthens individual systems and raises industry-wide awareness, establishing baseline protections against emerging threats.
However, identifying vulnerabilities is only the first step. Translating academic research into practical, deployable solutions is equally critical. For example, HiddenLayer’s SAI team has successfully adapted theoretical insights to address real-world security risks, demonstrating the importance of actionable research. By bridging the gap between theory and application, the industry can build scalable, adaptable defenses that protect AI systems and foster confidence in their use.
Building Safer AI Systems Through Innovation
Security must no longer be an afterthought in AI development—it must be integrated into every stage of the AI lifecycle. As AI technologies advance, so do the methods and motivations of attackers. Adversarial attacks, data poisoning, and other AI-specific threats are becoming more sophisticated, necessitating a shift toward 'secure by design' principles.
By embedding security into the development and deployment phases, organizations can mitigate risks before they materialize. This proactive approach not only reduces the likelihood of disruptions but also fosters trust in AI systems. As AI continues to transform industries, robust security measures will be essential to ensuring sustainable growth and safeguarding the integrity of these technologies.
Embracing security as a catalyst for responsible innovation will enable the development of resilient, reliable AI systems capable of withstanding evolving threats. This approach paves the way for future advancements that are both groundbreaking and secure, ensuring AI remains a force for positive change in an increasingly complex digital landscape.
Note: This publication was rewritten using AI. The content was based on the original source linked above.