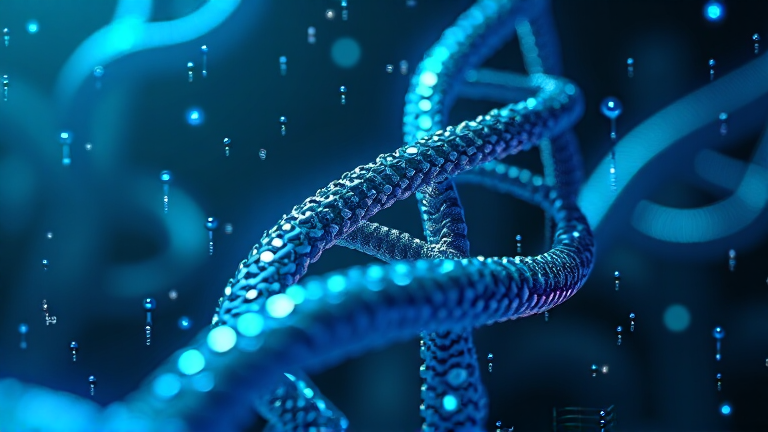
Overview
In a pioneering study, researchers demonstrated the use of the AI-enabled Pro-PRIME model to evolve a VHH antibody, enhancing its stability and resistance against extreme alkaline conditions. This achievement not only highlights the practicality of machine learning in protein engineering but also sets a new milestone in designing biotechnological tools for industrial mass production.
Concluding Insights
After two rounds of iterative design and rigorous experimental validation, the study confirmed that the Pro-PRIME model can reliably guide the engineering of proteins with unnatural traits. Notably, the enhanced VHH antibody exhibited improved thermal stability, robust alkali resistance, and maintained high binding affinity even after extensive cycles, thereby reducing production costs significantly. The integration of deep learning with rational design principles has broadened exploration possibilities beyond conventional methodologies, proving beneficial for industrial applications.
eLife Assessment
The work has been assessed as important with convincing evidence. Key findings include:
- Important: The study offers theoretical and practical insights that extend beyond a single subfield, contributing landmark advancements in protein engineering.
- Convincing: The use of robust, state-of-the-art methodologies provides solid backing for the claims made, affirming the efficacy of AI‐driven approaches over traditional design methods.
Learn more about eLife Assessments
Abstract
The research explores how artificial intelligence (AI) models can predict and engineer proteins that thrive in extreme industrial conditions. Conventional protein datasets often lack such extreme environments. Addressing this gap, the Pro-PRIME large language model was directed to evolve a VHH antibody for improved alkali resistance. Through the prediction of single- and multi-point mutations, two rounds of design resulted in mutants with higher thermal stability and enhanced affinity. The study marks the first instance where an AI-designed protein product has been successfully scaled for industrial mass production.
Introduction
Positioned at the intersection of molecular biology, bioinformatics, and biotechnology, protein engineering benefits greatly from computational advances. With the rise of deep learning, researchers have begun leveraging large-scale protein language models (LLMs) to expedite the design process. Despite encouraging results in optimizing properties such as thermostability and enzymatic activity, a major challenge remains—designing proteins that maintain functionality in extreme pH or saline conditions, traits typically absent in nature.
Historically, rational design and high-throughput screening were adopted to enhance protein resistance. However, these methods often result in significant trade-offs and are labor-intensive. In contrast, the Pro-PRIME model exploits deep learning to predict and subsequently validate both single-point and multi-point mutations, thereby overcoming limitations of conventional approaches. This study focuses on a VHH antibody engineered for binding growth hormone, aiming for enhanced alkali resistance while also improving thermal stability and affinity.
Experimental Design and Results
Single-Point Mutation Evaluation
Traditionally, protein engineering relies on identifying beneficial single-point mutations before combining them. In this study, the Pro-PRIME model generated zero-shot predictions for saturated single-point mutations of the VHH antibody. Out of the top 45 predicted mutations:
- 15 mutants showed improved alkali resistance (measured by lower half maximal effective concentration, EC50).
- 35 mutants demonstrated higher melting temperatures (Tm).
- 8 mutants achieved improved binding affinity compared to the wild type (WT).
Notably, six mutations (e.g., A57D, A15P) improved all three parameters, while a second set of eight mutations traded a slight cost in affinity for greater alkali resistance and thermal stability. Follow-up tests in harsher 0.5 M NaOH conditions validated that selected mutants performed significantly better than the WT.
Figure 1 (with supplements) illustrates the experimental metrics comparing mutation performance.
Multi-Point Mutation Design
The combination of single-point mutations into multi-point mutants was addressed by a two-stage design process. First, the model scored all single-point mutations. Then, experts pooled promising candidates into a multi-point mutant library. This approach, limited to 30–50 selected mutations, efficiently narrowed down the vast design space. Fine-tuning the Pro-PRIME model with experimental data enabled the identification of 20 multi-point mutants that collectively outperformed the best single-point mutations regarding both alkali resistance and thermal stability. For instance, a specific multi-point mutant improved alkaline resistance by 67.7% and increased melting temperature by over 10°C compared to the WT.
The study also emphasizes the critical role of deep learning: whereas traditional greedy algorithms risk falling into local optima, Pro-PRIME was able to incorporate even negative single-point mutations to produce unexpectedly beneficial multi-point combinations. This is evidenced by cases where combinations such as A57D;P29T exhibited properties far exceeding the sum of their individual effects.
Exploring Epistatic Effects
Further analysis revealed complex epistatic interactions. Two interesting cases include:
- Double Negative Yielding Positive: Two mutations that individually diminished performance combined to significantly enhance properties.
- Trade-offs in Combination: Some high-performing single-site mutations, when combined, resulted in reduced benefits.
These findings underscore the model's ability to probe non-linear interactions within the protein structure, which traditional design methods might miss. Figures 2 and 3 detail the epistatic effects and provide structural insights through SDS-PAGE analysis, sequence alignment, and molecular dynamics simulations.
Rational Design Revisited
The study juxtaposed AI predictions with traditional rational design principles. SDS-PAGE and mass spectrometry identified key cleavage sites that aligned with several of the top-performing mutations. Interestingly, while some mutations improved stability by aligning with consensus sequences, others defied conventional wisdom yet contributed positively when aggregated. Molecular dynamics simulations further corroborated that enhanced hydrogen bonding and overall structural rigidity were correlates of improved alkali resistance.
Broader Impact: Acid and Salt Resistance & Industrial Application
The improved VHH antibody also demonstrated exceptional resilience to acid and saline conditions. Figure 4 presents the performance under these stresses, showing that mutants could sustain high binding affinity even after treatment with acidic (ethanoic acid) or high-salt (NaCl) environments.
The practical application of the designed mutants is striking. After over 140 cycles of affinity chromatography, the engineered VHH antibody maintained up to 90% of its residual dynamic binding capacity (DBC), in stark contrast to the rapidly diminishing performance of the WT. This stability has already translated into industrial success, with the mutant being deployed in large-scale production (up to 5000 liters) and lowering manufacturing costs by more than one million dollars annually.
Discussion
By harnessing the power of the Pro-PRIME model, the research team has successfully pushed the boundaries of protein engineering to include properties rarely observed in nature. The two-round design process, which combined zero-shot predictions for single-point mutations with fine-tuned multi-point mutation strategies, resulted in a VHH antibody that meets diverse industrial demands, including exceptional alkali, acid, and salt resistance along with enhanced thermal stability and binding affinity.
The study not only underscores the potential of AI-assisted protein evolution but also demonstrates how deep learning models can unearth novel mutational combinations that transcend conventional rational design approaches. This breakthrough opens new avenues in medicine, agriculture, and bioengineering, where tailor-made proteins can be designed with specific, high-performance characteristics.
Materials and Methods
The article details several protocols to support reproducibility in the following key areas:
-
Prediction with Pro-PRIME Model: Utilizes masked language modeling and multi-task learning based on a vast sequence dataset. Techniques for both zero-shot screening and fine-tuning with experimental data are described.
-
Wet-Lab Procedures: Encompass plasmid construction, protein expression, purification, and various assays (e.g., ELISA for affinity tests and differential scanning fluorimetry for thermal stability).
-
Molecular Dynamics Simulations: Leverage AlphaFold3 for structure prediction and use state-of-the-art simulation packages (GROMACS 2020.4) to analyze dynamic stability and intramolecular interactions.
Detailed protocols for these methods are available through linked resources.
Data Availability and References
All data analyzed during this study are included in the manuscript and supplementary materials. The article cites foundational work in molecular simulations, protein design, deep learning applications, and rational engineering approaches.
For a complete list of references and additional technical details, readers are encouraged to consult the original documentation and supplementary protocols provided via external links.
Author Contributions, Funding, and Acknowledgements
The project was a collaborative effort among experts in physics, life sciences, applied mathematics, artificial intelligence, and medicine. Funding came from numerous prestigious institutions, and all contributors were duly acknowledged for their roles in conceptualization, experimentation, data analysis, and manuscript preparation.
Final Remarks
This work exemplifies how AI-driven strategies can revolutionize the field of protein engineering, not only by expediting the design process but also by broadening the scope of achievable protein modifications. The successful application of the engineered VHH antibody in large-scale industrial production positions the Pro-PRIME model as a transformative tool for future biotechnological innovations.
Note: This publication was rewritten using AI. The content was based on the original source linked above.