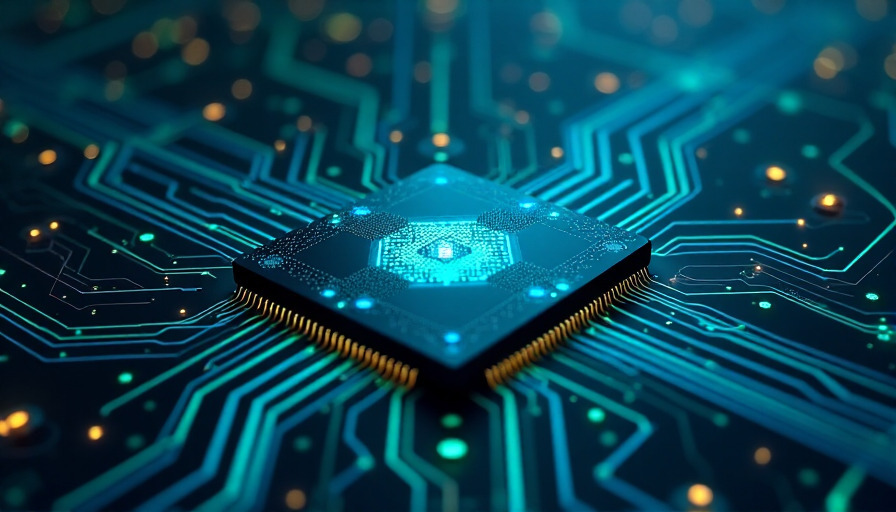
Meta Tests Its First In-House AI Training Chip
In a significant shift within the AI landscape, Meta has embarked on testing its very first homegrown chip designed specifically for AI training. This groundbreaking development marks Meta’s strategic move away from its heavy reliance on NVIDIA, which has long dominated the market for AI training chips.
A New Chapter in AI Hardware
Meta’s journey into developing custom AI chips is not new. The company has been working on bespoke designs like the “MTIA” and is planning to roll out its custom solutions in its data centers as early as the second half of 2024. The latest initiative, often referred to as the beginning of the "tape-out" phase, signifies that the chip design has already been forwarded to TSMC for manufacturing—a pivotal step in moving from concept to production.
Key Technological Insights
- Optimized for AI Tasks: The newly introduced chip is engineered as an AI accelerator, offering enhanced power efficiency compared to traditional GPU usage. This efficiency is crucial as it aims to handle AI workloads with speed and lower energy consumption.
- RISC-V Based Architecture: According to industry reports, this chip may utilize the RISC-V architecture, potentially setting a new industry precedent when combined with Meta's collaborative efforts with Broadcom and TSMC.
- Competitive Edge: Should the testing phase prove successful, Meta’s accelerator could vie with NVIDIA’s H200, B200, and even the next-generation B300 chips, achieving a performance that further diminishes the need for dependence on established chip manufacturers.
Challenges and Future Prospects
The process of bringing a chip from design to functional hardware is both costly and time-consuming. With tape-out expenses running into tens of millions of dollars and a timeline of three to six months, Meta faces a critical testing period. If the current prototype meets performance benchmarks, mass production will likely follow. However, any shortcomings during testing could necessitate a complete redesign, forcing Meta to revisit its approach.
Industry Impact and Broader Implications
This venture is not only a technological milestone for Meta but also a potential game changer in the AI hardware industry. By decreasing reliance on third-party chip manufacturers like NVIDIA, Meta could redefine cost structures, scalability, and efficiency in AI training processes worldwide. Industry experts speculate that such innovations might soon become the norm, spurring more companies to invest in custom-designed hardware.
Meta’s bold step into the realm of in-house chip design epitomizes the evolving narrative of technological self-reliance and innovation. The coming months will be critical in determining whether this pioneering effort can deliver on its promise of performance and efficiency, ultimately influencing the future dynamics of the AI hardware market.
Note: This publication was rewritten using AI. The content was based on the original source linked above.